What if the biggest risk to your cloud environment wasn’t a misconfiguration you made, but one baked into the defaults?
Our research uncovered security concerns in the deployment of resources within a few AWS services, specifically in the default AWS service roles. These roles, often created automatically or recommended during setup, grant overly broad permissions, such as full S3 access. These default roles silently introduce attack paths that allow privilege escalation, cross-service access, and even potential account compromise.
We found these flaws across several AWS services, including SageMaker
, Glue
, and EMR
, as well as in popular open-source projects like Ray
. These roles, originally intended for narrow, service-specific use, can instead be abused to perform administrative actions and break isolation boundaries between services.
In this blog, we’ll walk through multiple real-world scenarios, including how a malicious Hugging Face model can escalate privileges, how limited Glue
access can impact other services, and how a single default role can ultimately lead to full control of an AWS account.
We responsibly disclosed these issues to AWS, who responded promptly by adjusting default policies and publishing new security guidance.
Audit your AWS roles and restrict overly permissive access – especially to S3. Broad permissions in default roles can silently expose critical services across your environment.
What Are Default Roles in AWS?
AWS IAM (Identity and Access Management) controls who can access AWS resources and what actions they can perform. It links users, roles, and policies to services to manage permissions securely. When users first interact with an AWS service a default role is recommended or automatically created by AWS. The goal is to help users by offering IAM roles with pre-attached managed policies designed to a specific service or functionality on AWS. For example, when a user first accesses AWS Glue through the Management Console, a default role AWSGlueServiceRole
is automatically created.
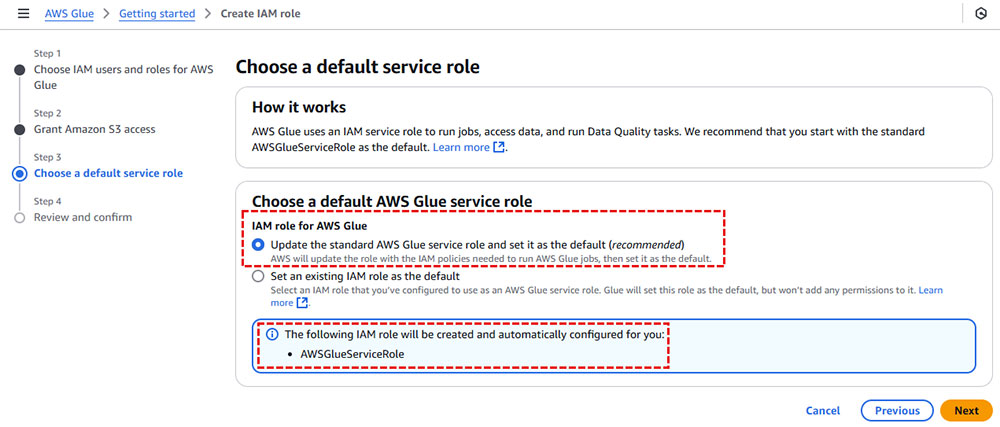
AWS Glue, by default, creates a default service role named AWSGlueServiceRole with pre-attached policies
In some of the cases we analysed, these default roles were granted overly broad access, such as the AmazonS3FullAccess
managed policy. When over-permissive policies are attached to default roles they can pose significant security risks.
As we’ll demonstrate in this blog, they can allow attackers to take over other services and, in some cases, escalate privileges to an admin role.
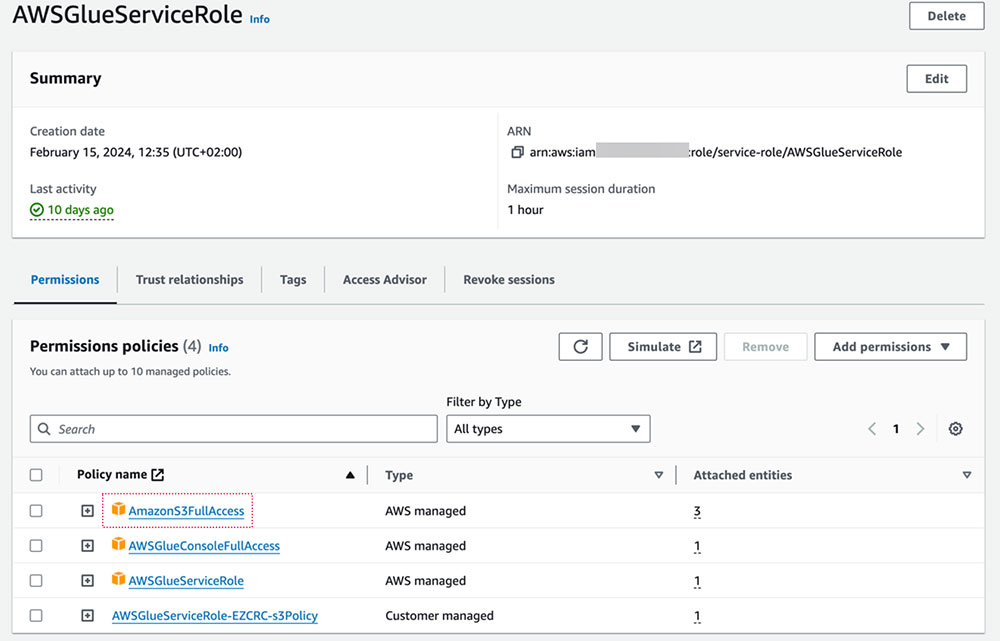
The default AWSGlueServiceRole had the AmazonS3FullAccess policy attached
The Risk of a AmazonS3FullAccess policy
While giving a role access to all S3 buckets with AmazonS3FullAccess
already sounds risky, the true impact is often underestimated. This permission doesn’t just expose stored data – it can allow attackers to tamper with other AWS services.
Why is this so dangerous?
Many AWS services rely on S3 to store essential assets like scripts, configuration files and templates. Gaining full access to S3 allows an attacker to manipulate the internal behaviour of services like CloudFormation, SageMaker, Glue, EMR, as well as tools like the AWS CDK
– escalating their privileges far beyond the original scope of the role.
We previously demonstrated at Black Hat USA 2024 and DEF CON 32 how predictable S3 bucket names could be exploited for remote code execution. In this case, attackers who gains access to a default service role with AmazonS3FullAccess
doesn’t even need to guess bucket names remotely. They can use their existing privileges to search the account for buckets used by other services, modify assets like CloudFormation templates, EMR scripts, and SageMaker resources, and move laterally across services within the same AWS account.
Several AWS services use predictable S3 bucket naming patterns:
- CloudFormation uses bucket with the pattern:
cf-templates-{Hash}-{Region}
- AWS CDK uses staging bucket with the pattern:
cdk-{qualifier}-assets-{account-ID}-{Region}
- SageMaker AI uses bucket with the pattern:
sagemaker-{Region}-{Account-ID}
- EMR uses bucket with the pattern:
aws-emr-studio-{Account-ID}-{Region}
- Glue uses bucket with the pattern:
aws-glue-assets-{Account-ID}-{Region}
With S3 full access, an attacker can easily locate these buckets, inject malicious content, and compromise other services.
In short – If any role in your account has AmazonS3FullAccess (either through an attached policy or inline permissions), it effectively has read/write access to every S3 bucket – and by extension, the ability to tamper with multiple AWS services. This turns a seemingly limited role into a powerful pivot point for lateral movement and privilege escalation within your cloud environment.
Exposing Default Roles with AmazonS3FullAccess
During our research, we found several default AWS service roles with overly broad permissions, often including the AmazonS3FullAccess
policy. Here are a few examples:
- Amazon SageMaker AI: SageMaker offers two ways of creating a domain (Single user or Quick Setup and Setup for Organizations). When setting up a SageMaker Domain under the Single user or Quick Setup an execution role named
AmazonSageMaker-ExecutionRole-<Date&Time>
is automatically created. This role comes with a custom policy equivalent to AmazonS3FullAccess (s3:GetObject
ands3:PutObject
permissions toarn:aws:s3:::*
). As a result, SageMaker notebooks have broad S3 access by default. - AWS Glue: The default
AWSGlueServiceRole
is created with theAmazonS3FullAccess
policy, granting extensive permissions to Glue jobs. - Amazon EMR: The default
AmazonEMRStudio_RuntimeRole_<Epoch-time>
role is automatically assigned theAmazonS3FullAccess
policy, allowing EMR notebooks to run with full access to S3.
We also saw some reference in the Amazon Lightsail AWS documentation that instructs users to attach the AmazonS3FullAccess
policy to a role or user to support the WordPress Offload Media plugin.
Exploiting Default AWS Roles: Attack Scenarios
The core idea behind this privilege escalation technique is simple: it depends on the service the attacker (or user) initially has access to.
For example, if an attacker gains access to a compromised role with permissions only for AWS Glue, they could create a new Glue job and modify its script to access additional S3 buckets (Glue jobs typically run using the default service role).
In the SageMaker AI case, the attacker would open a Jupyter notebook running under the SageMaker execution role and escalate privileges directly from the notebook. The same approach applies to EMR, where the attacker could open an EMR notebook running under the EMR runtime role and escalate privileges from there.
From there, the attacker can target S3 buckets linked to other AWS services. For example, they could look for buckets starting with cf-templates-*
(used by CloudFormation), inject a malicious CloudFormation template, and escalate privileges further.
Similarly, an attacker could target services like EMR, SageMaker by tampering with their associated S3 buckets. By modifying assets in these buckets, the attacker can run code in the context of the targeted service’s execution role, enabling lateral movement across the environment. In some cases, such as with AWS CDK or CloudFormation templates stored in staging S3 buckets are typically deployed with administrative permissions. If an attacker compromises a CDK staging bucket – whether through Glue, SageMaker AI, or EMR – and a user later deploys from it, the attacker can gain administrative access to the victim’s AWS account.
In the next sections, we’ll demonstrate two scenarios of this with SageMaker AI and AWS Glue. To better understand how attackers can target service buckets in general, refer to our previous research Bucket Monopoly: Breaching AWS Accounts Through Shadow Resources
Scenario 1: From SageMaker AI to Glue Service Takeover
In this scenario we show that by simply importing a malicious model from Hugging Face into SageMaker we could silently trigger code execution under a highly privileged role, putting the entire cloud environment at serious risk.
The Amazon SageMaker AI execution role includes a managed policy that allows ListBucket, PutObject and GetObject permissions for any S3 bucket in the account.
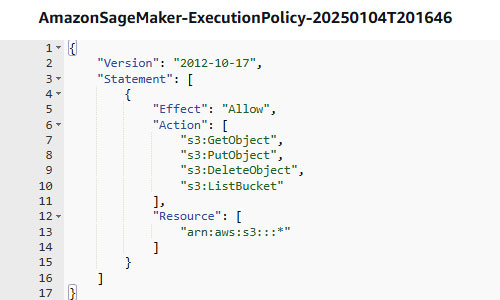
Policy attached to the default SageMaker execution role, granting near-full S3 access across all buckets – almost equivalent to AmazonS3FullAccess
An attacker could upload a malicious model to Hugging Face to target AWS SageMaker AI users.
When a Hugging Face model includes an inference.py
file with key functions like model_fn
(used to load the model) and predict_fn
(used to perform predictions), these functions are automatically invoked when SageMaker loads and uses a model.
If a SageMaker user imports malicious model from Hugging Face, SageMaker will execute the code inside inference.py during the model loading process. Specifically:
- When the model is loaded, SageMaker calls the
model_fn
function. - When a prediction is triggered, SageMaker calls the
predict_fn
function.
As seen in the screenshot above, a PoC of a malicious Hugging Face model containing an inference.py
file with model_fn
and predict_fn
. The attacker scans for Glue asset buckets and injects a backdoor into Glue job scripts to steal the IAM credentials of the Glue job when executed.
In the figure below you can see that, SageMaker automatically executes inference.py, even if trust_remote_code=False
, because the flag only controls Hugging Face’s Transformers loading – not SageMaker’s model serving behaviour. This means that even if users think they are disabling remote code execution by setting trust_remote_code=False
, SageMaker will still automatically run the inference.py file during model deployment.
Running untrusted models is inherently risky, but the danger is even greater because SageMaker operate under default execution roles with broad permissions, such as AmazonS3FullAccess
.
As a result, once a malicious model is loaded, the attacker’s code could modify assets belonging to other AWS services (such as CloudFormation templates or CDK deployment files).
As indicated in the above screenshot, the attacker’s code was executed and injected a backdoor into every aws-glue-assets-
bucket in the account. This was possible because the SageMaker execution role had permissions to upload to any S3 bucket in the account
Scenario 2: Escalating from Glue to Admin Access
An attacker who gains access to a role with AWS Glue permissions (such as glue:CreateJob
, glue:UpdateJob
, glue:StartJobRun
) and iam:PassRole
, or even a user with only the AWSGlueConsoleFullAccess
policy, can escalate their privileges within the account.
AWS Glue jobs typically run using the default AWSGlueServiceRole
, which included the overly permissive AmazonS3FullAccess
policy. By creating or modifying Glue jobs that run under this role, attackers can easily pivot beyond Glue, manipulate critical S3 buckets used by other services, and ultimately compromise the entire AWS environment.
Possible Attack Scenario:
- Initial Access: An attacker or user gains access to a role with permission to edit and run AWS Glue jobs. Typically, obtaining this role requires broader access beyond a single role, making this scenario more realistic for an insider or a user already present in the environment seeking to escalate their privileges.
- Glue Job Modification: The attacker edits an existing Glue job that already uses the default role AWSGlueServiceRole, or creates a new job, assigning it the
AWSGlueServiceRole
. - Overly Permissive Role: By default, the
AWSGlueServiceRole
has theAmazonS3FullAccess
policy attached, granting broad access to all S3 buckets in the account. - S3 Bucket Enumeration: The Glue job can then list all buckets in the account.
- Targeted Buckets: Some buckets are related to other AWS services. The attacker can search for buckets with specific prefixes, such as
cf-templates-*
for CloudFormation templates orcdk-*
for CDK assets. - Resource Injection: The Glue job is configured to continuously scan the account’s S3 buckets for new CloudFormation templates. When a new template is detected, the job modifies it before the stack creation process completes, injecting a malicious resource into the template. Since CloudFormation stacks are often deployed by privileged users through the management console, the attacker can exploit this opportunity to inject a new IAM role with administrative permissions (whoever initiated the CloudFormation service needs to have permissions to manage IAM role). Additionally, by focusing on CDK staging buckets (
cdk*
), the attacker can increase the chances of injecting an admin role, as CloudFormation templates stored in these buckets are typically deployed with elevated privileges.
Default Roles in Open-Source Projects
During our research, we found that this attack vector impacts not only AWS services, but also many open-source projects commonly used by organizations to deploy resources into their AWS environments. When deploying infrastructure with IaC tools like Terraform, Python (boto3)
libraries, and others, it is common for the deployment process to create default IAM roles to operate the project.
In many cases, these roles are configured with overly broad permissions – often attaching policies like AmazonS3FullAccess
, or similar permissions that allow full access to all S3 buckets in the account.
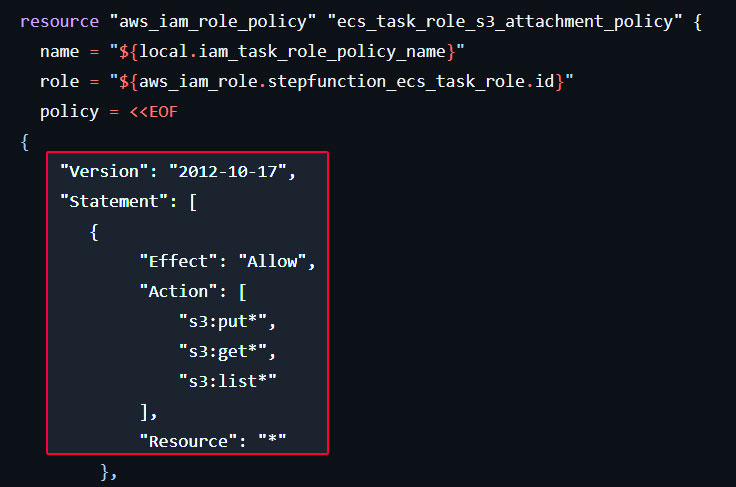
An example of a Terraform file that defines a policy allowing Put and Get permissions to every S3 bucket in the account
As part of our research, we found that Ray, a popular open-source framework, with over 36K start, for distributed computing and scaling machine learning workloads across cloud environments like AWS, automatically creates a default IAM role (ray-autoscaler-v1) with the AmazonS3FullAccess
policy hardcoded in its source code.
This grants full access to all S3 buckets in the AWS account, meaning an attacker who compromises the Ray EC2 instance could escalate privileges by manipulating services/projects that rely on S3 buckets behind the scenes, such as Glue, CloudFormation, EMR, CDK, SageMaker, and more – and potentially take over the entire AWS account. The Ray example is just the tip of the iceberg.
The example we provided highlights only one case among many. There are numerous projects that automatically create IAM roles and attach the AmazonS3FullAccess
policy, or similarly broad inline policies. Often, this is done to avoid breaking functionality or for user convenience, so that users don’t have to manually select specific S3 buckets required by the project. However, this widespread practice can put entire AWS environments at serious risk.
Disclosure
We contacted AWS to report the risks we identified in several default service roles. AWS responded quickly and took significant mitigation steps:
- Amazon SageMaker scoped down the default role for S3 buckets created during the setup process.
- AWS Glue restricted permissions granted during role creation and updated their documentation to educate users about enforcing the principle of least privilege.
- Amazon EMR scoped down the role for the S3 bucket that is configured during Studio creation.
- Amazon Lightsail updated its documentation to no longer instruct users to assign the AmazonS3FullAccess policy. Instead, users are now guided to create a specific bucket with a scoped-down policy for the WordPress Offload Media plugin.
In addition to these changes, AWS proactively sent notification emails to affected users, informing them that action is required to properly scope the permissions of their current default roles.
Separately, we also attempted to contact the Ray project security team to report similar issues in their deployment defaults. At the time of writing, we have not received a response.
AWS confirmed AWS CDK (Cloud Development Kit), AWS Glue, Amazon EMR (Elastic MapReduce), and Amazon SageMaker are operating as expected.
This issue was resolved by modifying the policies for the default service roles, particularly the AmazonS3FullAccess policy.
Amazon Lightsail has updated the documentation to instruct users to create buckets with a scoped-down policy.
AWS CDK has ensured CDK assets are only uploaded to buckets in the user’s account.
AWS provides customers with additional resources for the above services as they relate to the topics described in the blog:
- CDK: https://docs.aws.amazon.com/cdk/v2/guide/best-practices-security.html
- Glue: https://docs.aws.amazon.com/glue/latest/dg/security_iam_service-with-iam.html#security_iam_service-with-iam-roles-service
- EMR: https://docs.aws.amazon.com/emr/latest/ManagementGuide/emr-studio-service-role.html#emr-studio-service-role-serverless
- SageMaker: https://docs.aws.amazon.com/sagemaker/latest/dg/onboard-quick-start.html
- Lightsail: https://docs.aws.amazon.com/lightsail/latest/userguide/amazon-lightsail-connecting-wordpress-to-amazon-s3.html
We would like to thank Aqua Security for collaborating with us on this research through the coordinated disclosure process.
Summary and Mitigations
In this research, we uncovered flaws in several default IAM roles.
Overly permissive policies, particularly the assignment of AmazonS3FullAccess
, allowed roles intended for limited use to escalate privileges, manipulate other AWS services, and, in some cases, fully compromise AWS accounts.
Default service roles must be tightly scoped and strictly limited to the specific resources and actions they require. Organizations should proactively audit and update existing roles to minimize risk, rather than relying on default configurations.
To help mitigate these risks, we would like to offer a few recommendations:
- Avoid attaching
AmazonS3FullAccess
or any overly broad S3 permissions to service roles. - Always restrict S3 access to only the specific buckets required by the service.
- Regularly audit IAM roles and enforce the principle of least privilege to minimize the risk of privilege escalation and account compromise.
Aqua Platform users can leverage the ‘IAM Role Policies’ plugin as part of their security scans to detect overly permissive IAM configurations.
This plugin analyses both managed and inline policies attached to IAM roles and flags potential security risks. Specifically, it alerts users when:
- Managed policies grant broad permissions, such as allowing actions on all resources (e.g.,
"Resource": "*"
), which can open the door to unintended access across the environment. - Inline policies include wildcard actions like
s3:*
, which may unintentionally allow full access to sensitive services such as Amazon S3.
By identifying these misconfigurations early, Aqua helps teams enforce the principle of least privilege, reduce the attack surface, and strengthen their cloud security posture.